The Promising Role of Artificial Intelligence in Enhancing Diagnostic Accuracy in Medical Imaging
In recent years, the field of medical imaging has witnessed remarkable advancements, particularly in the realm of artificial intelligence
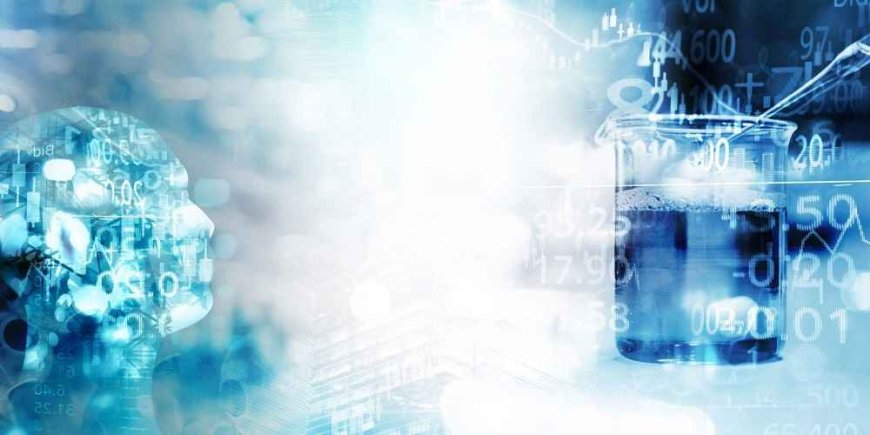
In recent years, the field of medical imaging has witnessed remarkable advancements, particularly in the realm of artificial intelligence (AI) and machine learning (ML). These technologies have shown tremendous potential in revolutionizing the diagnostic process, aiding clinicians in making accurate and timely decisions. With a particular focus on my medical and clinical interests, this article delves into the various ways AI is transforming medical imaging and enhancing diagnostic accuracy. By harnessing the power of AI, healthcare professionals can achieve improved patient outcomes and optimize resource allocation.
AI-Powered Image Analysis:
One of the primary applications of AI in medical imaging is image analysis. Convolutional Neural Networks (CNNs), a type of deep learning model, have demonstrated exceptional performance in identifying and classifying medical images. With large datasets and powerful algorithms, AI can quickly analyze images for the presence of abnormalities or diseases, thereby assisting radiologists in their diagnostic interpretation. For instance, AI algorithms can detect lung nodules in chest X-rays or identify suspicious lesions in mammograms, providing valuable support to clinicians.
Computer-Aided Detection and Diagnosis:
AI algorithms can also act as reliable computer-aided detection (CAD) systems, augmenting the capabilities of radiologists. By analyzing medical images alongside radiologists, AI can flag potentially problematic areas that may have been overlooked, thereby reducing the chances of missed diagnoses. Moreover, AI systems can help quantify and measure specific features, aiding in the assessment of tumor size, growth rates, or the progression of disease over time. This integration of AI into the diagnostic workflow ensures more accurate and comprehensive evaluations.
Predictive Analytics and Risk Stratification:
Beyond image analysis, AI can leverage patient data to perform predictive analytics and risk stratification. By integrating electronic health records (EHRs), genetic information, and imaging data, AI models can identify patterns and risk factors associated with certain diseases. This enables the development of personalized risk assessment tools, helping clinicians identify high-risk patients who may require closer monitoring or preventive interventions. AI-driven risk stratification can contribute to early detection and intervention, ultimately leading to better patient outcomes.
Precision Medicine and Treatment Planning:
AI holds great promise in the field of precision medicine, where treatment strategies are tailored to individual patients based on their unique characteristics. By analyzing vast amounts of patient data and medical literature, AI algorithms can provide insights into treatment response prediction, optimal drug dosages, and potential adverse reactions. This assists clinicians in making informed decisions, optimizing treatment plans, and reducing trial-and-error approaches. Furthermore, AI can facilitate the identification of novel therapeutic targets, leading to the development of more effective treatment options.
Workflow Optimization and Efficiency:
Implementing AI in medical imaging can enhance workflow efficiency and alleviate the burden on healthcare professionals. For instance, AI algorithms can prioritize image interpretation based on the urgency of cases, ensuring critical conditions receive immediate attention. Additionally, AI can automate routine tasks, such as image pre-processing or report generation, freeing up time for radiologists to focus on complex cases and direct patient care. By streamlining processes, AI can contribute to reduced waiting times, improved patient satisfaction, and increased productivity within healthcare settings.
Quality Assurance and Error Reduction:
Medical imaging is not immune to human error, and misinterpretations or missed findings can have significant consequences for patient care. AI can play a crucial role in quality assurance by acting as a second set of eyes, double-checking radiologists' interpretations. AI algorithms can compare radiologists' reports with the actual images, flagging discrepancies or potential errors for review. This iterative feedback loop between AI and radiologists helps improve accuracy and reduce diagnostic errors, ultimately enhancing patient safety.
Resource Allocation and Optimization:
Healthcare systems often face resource constraints, including a limited number of radiologists and increasing imaging volumes. AI can assist in optimizing resource allocation by triaging cases based on urgency and complexity. AI algorithms can analyze the severity of conditions, prioritize critical cases, and allocate radiologists' time accordingly. By ensuring that high-priority cases receive prompt attention, AI helps expedite diagnosis and treatment, leading to improved patient outcomes and enhanced overall healthcare efficiency.
Continuous Learning and Improvement:
One of the unique aspects of AI is its ability to continuously learn and improve over time. By leveraging large-scale datasets and feedback from radiologists, AI algorithms can refine their performance and adapt to evolving clinical practices. As more data becomes available, AI systems can incorporate new knowledge and refine their diagnostic capabilities. This iterative process of learning ensures that AI remains up to date with the latest advancements and contributes to ongoing improvements in diagnostic accuracy.
Ethical Considerations and Transparency:
As AI becomes more integrated into medical imaging, it is essential to address ethical considerations and ensure transparency in its use. Clear guidelines and regulations should be established to govern the development, validation, and deployment of AI algorithms. Ethical issues such as patient privacy, data security, and bias detection and mitigation need to be carefully addressed to maintain patient trust and uphold the principles of medical ethics.
Collaborative Decision-Making:
While AI algorithms show great promise in enhancing diagnostic accuracy, they should be viewed as tools that augment human expertise rather than replace it. Collaborative decision-making, where radiologists and AI work together, can yield the best outcomes. Radiologists bring their clinical expertise and contextual understanding, while AI provides data-driven insights and assists in pattern recognition. This synergistic approach maximizes the potential of both human intelligence and AI technology, ensuring the best possible diagnostic outcomes for patients.
In conclusion, the integration of artificial intelligence in medical imaging holds immense potential for enhancing diagnostic accuracy and clinical decision-making. From quality assurance and error reduction to resource optimization and continuous learning, AI is poised to revolutionize the field. However, careful attention must be given to ethical considerations and transparency, and AI should be viewed as a collaborative tool rather than a replacement for human expertise. By harnessing the power of AI, healthcare professionals can unlock new possibilities, deliver personalized care, and improve patient outcomes in the realm of medical imaging.