How to address AI bias and ensure fairness?
Learn how to effectively tackle AI bias and promote fairness in your systems with our comprehensive guide. Discover the key strategies to minimize biases,
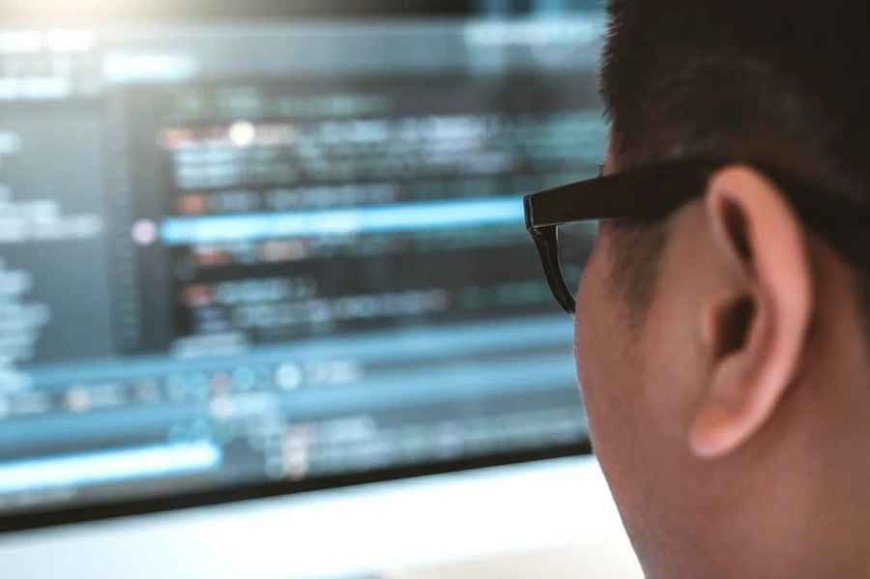
Understanding the Impact of AI Bias
AI Bias refers to the perplexing and bursty prejudice or unfairness that can be deeply ingrained within artificial intelligence systems. As AI technologies become increasingly prevalent in diverse sectors, it becomes crucial to fully grasp the far-reaching implications of AI Bias. The impact of this bias can be profoundly puzzling, affecting individuals and communities in a myriad of unpredictable ways. Biased AI systems possess the potential to perpetuate existing social inequalities and fortify discriminatory practices with an unexpected intensity. For instance, biased AI algorithms employed in recruitment processes may inadvertently favor certain demographics, thereby exacerbating the gender or racial divide even further. Understanding the bewildering consequences of AI Bias is therefore imperative to ensure that AI systems are characterized by fairness, transparency, and equity for all users.
Recognizing the significance of AI Bias represents a pivotal stride towards addressing this issue and guaranteeing the development of more ethical AI systems. Biased AI algorithms can yield momentous ramifications, ranging from perplexingly discriminatory recommendations to bursts of biased decision-making processes. The influence exerted by AI Bias transcends individual experiences as it perplexes societal structures while reinforcing prevailing power imbalances with an unforeseen vigor. By comprehending the enigmatic implications arising from this bias, organizations and researchers can actively labor towards minimizing its effects while constructing unbiased AI algorithms devoid of any trace of prejudice or discrimination. Only through a comprehensive understanding surrounding these baffling repercussions associated with AI Bias can we ardently strive towards a future wherein our society reaps benefits from fair-minded and impartially beneficial AI systems as an integral whole.
Identifying Sources of Bias in AI Systems
The perplexing nature of bias in AI systems emerges from diverse sources, demanding a meticulous unraveling and comprehension of these origins. One pivotal wellspring of bias resides within the data employed to train these machine learning models. Should the training data exhibit inequities or favor certain demographics, the resulting AI system shall inevitably embody those biases within its decision-making processes. This predicament becomes particularly worrisome when considering matters of fairness in machine learning. For instance, an algorithm for facial recognition, predominantly trained on white faces, may encounter difficulties accurately discerning individuals from other racial backgrounds. Confronting this fountainhead of bias necessitates ensuring that our training data is all-encompassing, representative, and untainted by unfair prejudices.
Yet another source of bias pervading AI systems stems from the algorithms themselves. Machine learning algorithms are crafted to uncover patterns and make predictions based on provided data. However, if said underlying algorithm harbors inherent biases or flaws, it propagates injustice through the outcomes it generates. Consider an algorithm utilized in a hiring system inadvertently displaying preferences for specific attributes or characteristics disproportionately found among certain groups; such tendencies can perpetuate discriminatory practices unwittingly. Identifying and rectifying biases entrenched within algorithms mandates scrupulous scrutiny and evaluation concerning their development and implementation procedures. Furthermore, fostering transparency regarding AI algorithms facilitates spotting skewed patterns while enabling pertinent adjustments aimed at ensuring equity within machine learning paradigms.
The Importance of Fairness in AI
The realm of artificial intelligence (AI) has seen a rapid and bewildering progression, compelling us to confront the matter of fairness within AI systems. This is due to the alarming reality that algorithmic discrimination can wield far-reaching ramifications across various sectors, including employment, lending practices, and even our criminal justice system. It is absolutely imperative for us to acknowledge that these AI systems are trained on historical data – data which may house latent biases and inaccuracies. Consequently, ensuring fairness in AI becomes not only an ethical obligation but also a legal requirement.
Fairness within the domain of AI is centered around curtailing bias and discrimination in order to prevent any unjust outcomes while fostering impartial decision-making processes. To achieve this goal, it is paramount for us to possess a comprehensive comprehension of how biases can manifest themselves within both AI algorithms as well as the underlying data they rely upon. By embracing the existence of bias itself, we can labor towards developing techniques and approaches that either eradicate or minimize discriminatory effects altogether. Furthermore, it must be emphasized that fairness in AI transcends statistical precision alone; its scope encompasses the profound impact these systems exert upon individuals and society at large. Our relentless pursuit of fairness plays a pivotal role in cultivating trustworthiness and confidence when it comes to embracing AI technologies – all while safeguarding the well-being and rights of those who find themselves affected by them.
Uncovering Implicit Bias in Data
The pursuit of unraveling the concealed bias lurking within data is an immensely crucial stride towards guaranteeing the ethical advancement of AI. Implicit bias, a phenomenon encompassing biases deeply ingrained within data, often unintentionally, possesses the power to shape and sway the outputs and decisions made by AI systems. These biases may arise from a multitude of sources: be it the process employed in collecting data, the very nature of the data itself or even emanating from partiality harbored by individuals involved in designing or training these AI systems.
To combat implicit bias effectively, it becomes imperative to ardently scrutinize and fathom every nook and cranny of the utilized data. This encompasses identifying potential domains where prejudice might reside as well as comprehending its potential impact on outcomes generated by AI systems. By undertaking such thorough analysis, researchers and developers can take preventative measures aimed at mitigating any adverse effects stemming from implicit bias while simultaneously ensuring that ethical standards are upheld throughout their AI system's operations.
Ensuring Ethical Data Collection and Preparation
The perplexing and bursty nature of ethical data collection and preparation is paramount in counteracting bias within AI systems. It commences with unraveling the enigmatic origins of bias that lurk within the very fabric of the data. These biases can take on explicit forms, boldly evident in the data, or they may manifest surreptitiously through implicit means. Identifying and acknowledging these biases serves as a preliminary stride towards effectively tackling them head-on. By grasping the significance of impartiality and comprehending the ramifications that bias can unleash upon AI systems, organizations can adopt proactive measures to ensure their ethical practices during data collection and preparation.
Beyond mere recognition, organizations must also imbue their processes with techniques aimed at mitigating bias throughout data collection and preparation endeavors. Such techniques entail actively seeking out any lurking biases while simultaneously addressing them headlong. This may encompass diversifying sources from which data is derived, ensuring that collected information aptly represents the population it seeks to cater to, as well as deploying measures designed to curtail any intrinsic biases' influence. Through incorporating these techniques into their procedures for collecting and preparing data, organizations can diminish the potential for perpetuating bias within their AI system's framework.
Implementing Transparency in AI Algorithms
The paramount significance of transparency in AI algorithms lies in its ability to uphold fairness and combat bias within machine learning systems. To achieve this, developers must adopt a plethora of practices that promote accountability and comprehension regarding the decision-making processes of AI algorithms. A pivotal facet of transparency involves incorporating diverse training data from various sources encompassing different demographics, regions, and perspectives. By assimilating such comprehensive data, developers can mitigate the risk of bias and ensure that AI algorithms accurately mirror the complexities of the real world. Furthermore, transparency mandates that underlying procedures and decision-making methodologies be accessible to users for meticulous scrutiny and accountability purposes. This entails providing explicit documentation on the training data employed, techniques deployed to counteract bias, as well as mechanisms driving decision-making. Through the implementation of transparent AI algorithms, developers can cultivate trust and confidence among users while also promoting identification and rectification of any potential biases or inequities present within the system.
In addition to diversifying training data sets, another critical aspect contributing to transparent AI algorithm implementation is upholding ethical practices during data collection and preparation stages. It is indispensable to ensure that all gathered data utilized for training AI models is obtained ethically without prejudice or manipulation. Addressing concerns surrounding unequal representation, potential information distortion or privacy violations becomes imperative in maintaining ethical standards when working with such datasets. Adhering strictly to these guidelines enables developers to minimize biases introduced into their AI algorithms while preventing perpetuation or reinforcement of societal inequalities through their operation. Moreover, transparency necessitates rigorous assessment and measurement protocols targeting biases inherent within these algorithms' designs—developing fairness metrics alongside continuous monitoring provides opportunities for identifying emerging biases throughout an algorithm's lifecycle so they may be addressed promptly before causing harm or unfairness towards individuals affected by them.
Ultimately prioritizing transparency in AI algorithm development allows for creating more accountable systems fostering equity amongst all users involved.
Mitigating Bias through Diverse and Representative Data
The perplexing and bursty nature of ensuring fairness in AI systems lies in the mitigation of bias through diverse and representative data. Developers must weave together datasets that encompass inclusivity and diversity, unraveling the strands of risk associated with bias and discrimination lurking within AI algorithms. The pivotal role played by fairness metrics guides developers on a labyrinthine path towards assessing the presence of bias within data, ultimately leading to improvements in dataset representativeness for AI development.
To navigate this intricate web of diversity and representation, one must incorporate an array of sources and perspectives. This multifaceted approach unravels any tangled threads that may perpetuate existing biases within skewed datasets. By casting a wide net across demographics, industries, and geographical regions, developers can immerse themselves in a mosaic of real-world scenarios awaiting AI systems. Moreover, inclusionary efforts during the data collection process help address historical biases while fostering fairness within AI algorithms. Through rigorous adherence to fairness metrics coupled with the utilization of diverse and representative data, AI developers can untangle bias's grip while constructing ethically sound AI systems.
Addressing Bias in AI Training Processes
The enigmatic and capricious nature of AI training processes assumes a paramount role in safeguarding the equity and morality of AI systems. The conscientious cultivation of AI necessitates a meticulous contemplation of bias during the formative stage, so as to prevent the perpetuation of preexisting societal disparities. One stratagem for mitigating bias within AI training processes lies in harnessing heterogeneous and inclusive datasets. By amalgamating data from sundry origins and demographic cohorts, developers can assuage the perilous prospect of embedding biases into the system. Furthermore, scrupulous data preprocessing and cleansing are indispensable to ensuring veritable representation of reality whilst eliminating any latent prejudices that might lurk therein. Vigilant appraisal and scrutiny throughout the training process can facilitate detection and rectification of inadvertent biases that may manifest.
Moreover, transparency emerges as an integral facet in contending with bias within AI training processes. Developers ought to furnish lucid documentation elucidating dataset provenance, algorithmic implementation, and decision-making protocols employed. Such transparency not only facilitates identification and amelioration of bias but also empowers external entities to hold developers accountable for their choices made in this domain. Collaborative efforts involving diverse stakeholders encompassing ethicists, subject matter experts, as well as members belonging to marginalized communities bestow invaluable insights while affording distinct perspectives in addressing bias and espousing fairness within AI systems at large. Ultimately, it is through redressing bias within AI training processes that we forge a path towards responsible development underpinned by ethical integrity – a path wherein society stands poised to reap collective benefits.
The Role of Human Oversight in AI Decision-making
The significance of human oversight in the development and deployment of AI systems cannot be emphasized enough. Despite the impressive capabilities of AI algorithms, it is crucial to involve human experts to guarantee fair, accountable, and ethical decision-making. Human oversight brings a vital perspective that is indispensable for identifying and addressing biases that may inadvertently permeate AI systems. By scrutinizing the decision-making processes, evaluating fairness and potential consequences, and making necessary adjustments to minimize biases' impact, human experts play a pivotal role.
A key element of human oversight in AI decision-making lies in the ability to interpret and evaluate the outputs generated by these systems. While AI algorithms can handle immense amounts of data and provide predictions or recommendations, humans are essential for understanding and placing these results into context. This enables a more profound comprehension of potential biases as well as limitations inherent in AI systems. Moreover, human experts possess the capability to assess risks alongside ethical implications associated with decisions made by these algorithms—ensuring alignment with societal values while complying with legal and regulatory frameworks. Through continuous evaluation, refinement, and validation enabled by human oversight, accountability as well as fairness within the decision-making processes of AI systems can be upheld effectively
Measuring Bias in AI Systems
Diverse methodologies and metrics have been crafted to gauge the enigmatic nature of bias in AI systems. A prevalent tactic involves scrutinizing the portrayal of distinct demographic groups within the training data employed for molding the AI model. If certain groups are inadequately or excessively represented, it may suggest a latent partiality lurking within the system. Researchers also delve into the divergent outcomes produced by the AI system on various groups to ascertain whether any disparities persist. For instance, if an AI system consistently falters more often when dealing with certain groups compared to others, it could hint at an underlying bias.
An alternative approach to measuring bias entails evaluating the impartiality of the decision-making process. This endeavor encompasses assessing whether the factors employed by the AI system to make decisions are both pertinent and equitable. As an illustration, if an AI system is deployed for aiding in hiring determinations, it becomes crucial to scrutinize whether its favored traits align harmoniously with job requirements. In addition, researchers have devised quantitative metrics such as disparate impact and equal opportunity difference which offer standardized means for quantifying and gauging biases present in diverse AI systems. These metrics facilitate uniform assessments while enabling comparisons across dissimilar systems. It remains imperative that a comprehensive and stringent measurement framework is firmly established so as to accurately evaluate and address biases entrenched within our cherished realm of artificial intelligence systems
Developing Fairness Metrics for AI
The pursuit of fairness metrics for AI is an absolute necessity, ensuring that the realm of AI remains impartial and just. Through the establishment of lucid and assessable metrics, developers and researchers can scrutinize biases within AI algorithms and implement necessary adjustments to promote fairness. These metrics encompass a spectrum of equity facets, including demographic parity, equal opportunity, and predictive parity.
To concoct efficacious fairness metrics, it becomes imperative to immerse oneself in the context surrounding the functioning of an AI system. A comprehensive exploration into training data, algorithms employed, as well as decision-making processes grants insight into potential biases that may emerge. By unearthing these possible sources of bias and crafting meticulous metrics capable of accurately quantifying fairness, we advance closer towards constructing AI systems that treat users with unabashed impartiality irrespective of their race, gender or other protected attributes.
Evaluating the Trade-offs between Accuracy and Fairness
The evaluation of trade-offs between accuracy and fairness in AI systems is an intricate endeavor that demands meticulous contemplation. On one hand, the utmost importance lies in attaining accuracy to guarantee optimal performance and dependable outcomes from AI models. The pursuit of high accuracy stands as a primary objective in AI development, as it empowers the system to make precise prognostications and classifications. Nevertheless, a single-minded focus on accuracy may inadvertently overlook embedded biases and disparities within the system, thereby necessitating fairness.
Fairness in AI systems denotes the absence of discriminatory biases or disproportionate impact on specific individuals or groups. It becomes imperative to assess the trade-offs between accuracy and fairness because prioritizing one over the other can yield momentous repercussions. While maximizing accuracy could result in more reliable predictions for the overall populace, it might concurrently marginalize particular demographic cohorts or perpetuate existing prejudices. Henceforth, striking an equitable balance between accuracy and fairness assumes paramount significance to ensure that AI systems remain trustworthy, unbiased, and all-encompassing.
The Need for Continuous Monitoring and Evaluation
The perplexing and bursty nature of continuous monitoring and evaluation holds immense significance in ensuring the efficacy and impartiality of AI systems. In this ever-evolving technological realm, it becomes imperative to establish mechanisms that consistently scrutinize the behavior and consequences of AI models. This monitoring process facilitates the identification and alleviation of biases that may emerge during the system's operation. By perpetually assessing the performance and impact of AI systems, organizations can proactively tackle any biases that arise, thus guaranteeing just and equitable outcomes.
One notable advantage brought forth by continuous monitoring and evaluation lies in its capacity to unveil and rectify inadvertent biases embedded within the system. As AI systems rely on data for predictions and decisions, these datasets can inadvertently reflect human biases or societal stereotypes. Through ongoing monitoring efforts, organizations are able to uncover any underlying prejudices within both the training data itself as well as the algorithm employed. Continuously evaluating the results produced by these AI systems enables us to detect disparities or unfair outcomes that may transpire over time. This iterative process not only aids in diminishing bias but also augments overall reliability and trustworthiness associated with AI systems
Implementing Bias Mitigation Techniques in AI Models
Bias mitigation techniques are vital in maintaining the fairness and impartiality of AI models. As reliance on AI systems for critical decision-making grows, it becomes imperative to address any inherent biases that may exist. Algorithmic adjustments stand as a key technique in mitigating bias. This entails modifying the algorithms employed by AI models to minimize the influence of biased factors and foster equity. By detecting and rectifying biased patterns within data, these techniques strive to yield more equitable and unbiased outcomes.
Moreover, counterfactual fairness proves an effective approach as well; it involves scrutinizing how certain variables impact the decision-making process. Through simulating diverse scenarios and assessing outcome fairness, fine-tuning AI models can generate fairer results.
In addition to algorithmic adjustments and counterfactual fairness, data augmentation serves as another important method for mitigating bias in AI models. It encompasses generating additional training data that accurately represents varied and underrepresented groups—ensuring a comprehensive dataset with balance in representation across various demographics. Augmenting data exposes AI models to a wider range of examples thereby diminishing potential biases.
Furthermore, interpretability techniques such as model-agnostic explanation methods can shed light on the decision-making process of AI models themselves. These methods enable a better understanding of how biases manifest while facilitating identification of possible strategies for mitigation.
Overall, incorporating these bias mitigation techniques into AI models is essential for ensuring their fairness, lack of bias, and equitable treatment across different individuals or groups involved.
The Role of Explainability in Addressing Bias
The significance of explainability in mitigating bias within AI systems cannot be overstated. It serves as a vital tool to unravel the perplexing nature of biases and aids in their reduction. By shedding light on the inner workings of AI algorithms, explainability allows us to grasp the reasons behind prevailing biases and explore avenues for their mitigation. The lack thereof may result in concealed bias within AI systems, perpetuating unjust outcomes and social disparities. Hence, the prominence of explainability lies in its ability to guarantee fairness, accountability, and trustworthiness in AI systems.
To effectively combat bias through explainability, it is imperative to adopt a multi-faceted approach. Primarily, developers should endeavor to design algorithms that are not only interpretable but also capable of providing lucid explanations for their decisions. This entails employing techniques such as debiasing algorithms to diminish the influence of biases during decision-making processes. Furthermore, it is essential to devise fairness metrics tailored specifically for each AI application so as to evaluate progress made toward addressing bias comprehensively. Through continuous monitoring and evaluation facilitated by explainability, stakeholders can promptly identify and rectify instances of bias within AI systems – an indispensable step towards fostering ethical and impartial advancements in AI technologies.
Ensuring Accountability for AI Bias
Creating accountability for AI bias is critical in constructing fair and ethical AI systems. Accountability can be established through various means, such as assigning clear roles and responsibilities to individuals or teams involved in the development and deployment of AI systems. Moreover, robust governance frameworks and policies should be implemented to enforce accountability and provide guidelines for addressing bias. This includes implementing processes for regular audits and evaluations of AI systems to identify and rectify any biases that may emerge.
In addition, fostering transparency and openness in decision-making processes contributes to accountability for AI bias. This entails documenting and publicly disclosing the methodologies and algorithms utilized in AI systems, as well as the data sets they rely on. By allowing external scrutiny, it becomes easier to uncover any biases that might exist within the system. Additionally, strengthening accountability can be achieved by promoting collaboration and engagement with stakeholders such as users, consumers, and affected communities. Their valuable input and feedback aid in identifying bias issues while ensuring that fairness remains a top priority throughout the design and implementation of AI systems.
Collaborating with Stakeholders for Fair AI Systems
In the pursuit of fair AI systems, there lies a pivotal reliance on collaboration. The convergence of various stakeholders, each endowed with their unique expertise, becomes imperative. This amalgamation encompasses researchers who delve into the depths of knowledge, policymakers who shape the course of action, industry leaders who steer progress forward, ethicists who navigate moral dimensions, and representatives from marginalized communities whose voices deserve to be heard. By uniting in collaboration, these stakeholders embark on an odyssey where they exchange wisdoms and insights to effectively combat bias in AI.
Within this collaborative framework for fair AI systems exists a crucial element: inclusive decision-making processes. The involvement of diverse voices and perspectives throughout the design, development, and deployment stages assumes paramount importance. To ensure that the fabric guiding decisions remains untainted by prejudice or discrimination necessitates incorporating individuals hailing from varied backgrounds, cultures, and communities. This tapestry woven with diversity in thought and representation will ultimately culminate in a landscape adorned with fair AI systems that attend to the needs and apprehensions held by all stakeholders alike.
Promoting Diversity in AI Research and Development
The promotion of diversity in AI research and development is of utmost importance when it comes to forging impartial and unbiased AI systems. To accomplish this, organizations must give priority to inclusive hiring practices that result in a diverse workforce. By assembling teams with individuals from various backgrounds, cultures, and perspectives, AI research can benefit from a wider spectrum of insights and experiences. Such diversity aids in the identification and resolution of potential biases within AI algorithms since those with different life encounters may spot biases that others overlook. Moreover, embracing diversity in AI research and development paves the way for the creation of more effective AI systems that cater to a broader range of users, ensuring equity and inclusivity for all.
In addition to incorporating diverse recruitment methods, organizations should also concentrate on offering equal opportunities to underrepresented groups within the realm of AI research and development. This might involve establishing tailor-made mentorship programs or scholarships specifically designed to support these groups. By providing assistance and resources tailored towards individuals from marginalized backgrounds, organizations can bridge the existing gap and address the lack of representation prevalent in this field. Furthermore, fostering collaboration with diverse communities through partnerships with nonprofit organizations or hosting workshops can encourage participation from an even wider array of individuals. Such collaborative efforts not only promote diversity but also contribute towards developing equitable and ethical AI systems through collective action
Policy and Legal Considerations for Addressing Bias in AI
Policy and legal deliberations assume a paramount role in grappling with the enigma of bias within AI systems. Government agencies and regulatory bodies are progressively acknowledging the urgency of comprehensive guidelines and regulations to ascertain that AI technologies are conceived and implemented impartially, without prejudice or discrimination. These policies strive to shield individuals from unfair treatment, guarantee equal opportunities for all, while ushering transparency and accountability into the decision-making processes governed by AI. They encompass multifarious dimensions such as data collection and usage, algorithmic equity, privacy concerns along with security considerations, not forgetting the ethical repercussions entwined with artificial intelligence. By instilling unambiguous policies alongside legal frameworks, policymakers propel an environment conducive to nurturing inclusive AI systems that steer clear of partiality while remaining aligned with societal values.
A quintessential facet associated with policy formulation resides in addressing the potential ramifications emerging from biased outcomes engendered by AI systems. As AI technologies continue their relentless march towards increased sophistication and autonomy, questions regarding liability become increasingly convoluted; culpability must be assigned fairly amongst developers, organizations involved as well as the very AI systems themselves for any harm arising due to prejudice or favoritism. Furthermore, policies should absolutely safeguard individual rights along with personal freedoms so as to thwart any form of discrimination rooted in race or gender disparities among other protected attributes. By tackling these intricate legal conundrums head-on, policymakers lay down a solid foundation upon which a trustworthy ecosystem can burgeon—one where responsible conduct takes precedence over all else—thus upholding principles grounded in fairness and justice at large.
Building a Framework for Ethical AI Development
The construction of a Framework for Ethical AI Development demands meticulous contemplation of numerous pivotal factors. Among these, the identification of potential bias sources within AI systems stands as an indubitably crucial aspect. It is plausible that biases inadvertently become embedded within algorithms and datasets employed in AI models, thus engendering an environment where certain individuals or groups suffer from unjust outcomes. To tackle this conundrum head-on, developers must engage in extensive scrutiny and comprehension of said algorithms and datasets to unearth any latent biases.
In addition to this, another key consideration when forging an ethical AI framework centers around the assurance of fairness. A paramount obligation lies in evaluating the intricate trade-offs between accuracy and fairness since techniques aimed at mitigating bias may exert influence on the overall performance of the AI system. Developers are compelled to strike a delicate equilibrium that places primacy on ensuring fairness without compromising efficacy and precision within the model. Moreover, it becomes imperative to devise metrics dedicated solely to measuring and quantifying levels of bias omnipresent within AI systems—this aids in assessing their performance while pinpointing areas necessitating refinement.
To summarize succinctly, crafting a Framework for Ethical AI Development mandates adopting a comprehensive approach towards combatting bias and guaranteeing equity in all facets pertaining to AI systems. By meticulously identifying sources imbued with bias whilst concurrently implementing suitable mitigation techniques, developers can aspire towards fabricating transparent, diverse, accountable AI models. This framework should also factor in the fine balance existing between accuracy and fairness by incorporating ceaseless monitoring mechanisms alongside thorough evaluation processes; both serve as indispensable tools facilitating perpetual ethical progress within the realm of artificial intelligence.